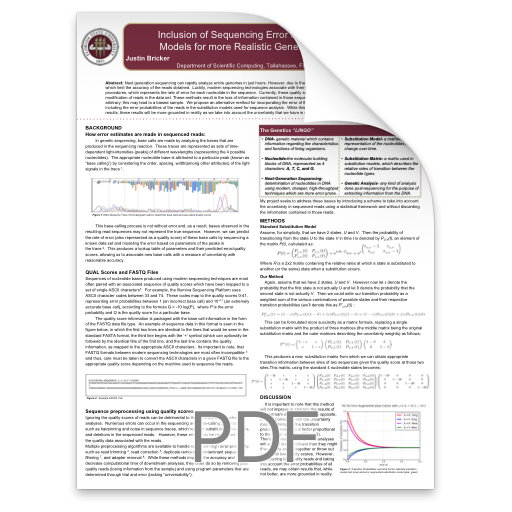
Authors
Title
Inclusion of Sequencing Error in Substitution Models for more Realistic Genetic Analyses
Abstract
Next generation sequencing can rapidly analyze entire genomes in just hours. However, due to the nature of the sequencing process, errors may arise which limit the accuracy of the reads obtained. Luckily, modern sequencing technologies associate with their reads, a quality score, derived from the sequencing procedures, which represents the rate of error for each nucleotide in the sequence. Currently, these quality scores are used as a criteria for the removal or modification of reads in the data set. These methods result in the loss of information contained in those sequences and rely on parameters that are somewhat arbitrary; this may lead to a biased sample. We propose an alternative method for incorporating the error of the sequences without discarding poor quality reads by including the error probabilities of the reads in the substitution models used for sequence analysis. While this method will result in analyses with less defined results, these results will be more grounded in reality as we take into account the uncertainty that we have in our sequenced samples.