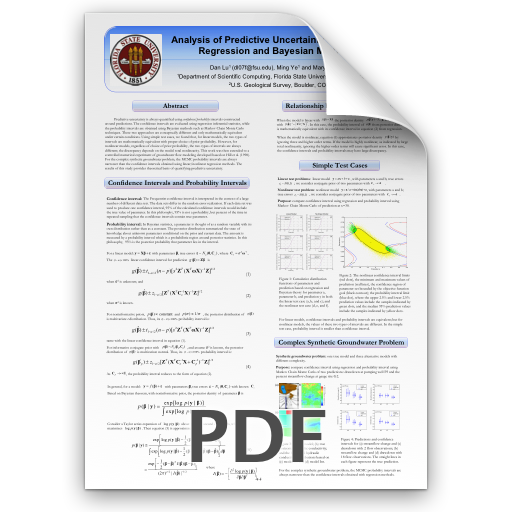
Analysis of Predictive Uncertainty Measures of Regression and Bayesian Methods
Abstract
Predictive uncertainty is always quantified using confidence/ probability intervals constructed around predictions. The confidence intervals are evaluated using regression inferential statistics, while the probability intervals are obtained using Bayesian methods such as Markov Chain Monte Carlo techniques. These two approaches are conceptually different and only mathematically equivalent under certain conditions. Using simple test cases, we found that, for linear models, the two types of intervals are mathematically equivalent with proper choice of prior probability. However, for nonlinear models, regardless of choice of prior probability, the two types of intervals are always different; the discrepancy depends on the model total nonlinearity. This work was then extended to a controlled numerical experiment of groundwater flow modeling developed based on Hill et al. (1998). For the complex synthetic groundwater problem, the MCMC probability intervals are always narrower than the confidence intervals obtained using linear/nonlinear regression methods. The results of this study provides theoretical basis of quantifying predictive uncertainty.